Managing Recycling Uncertainty with Data-Driven Models
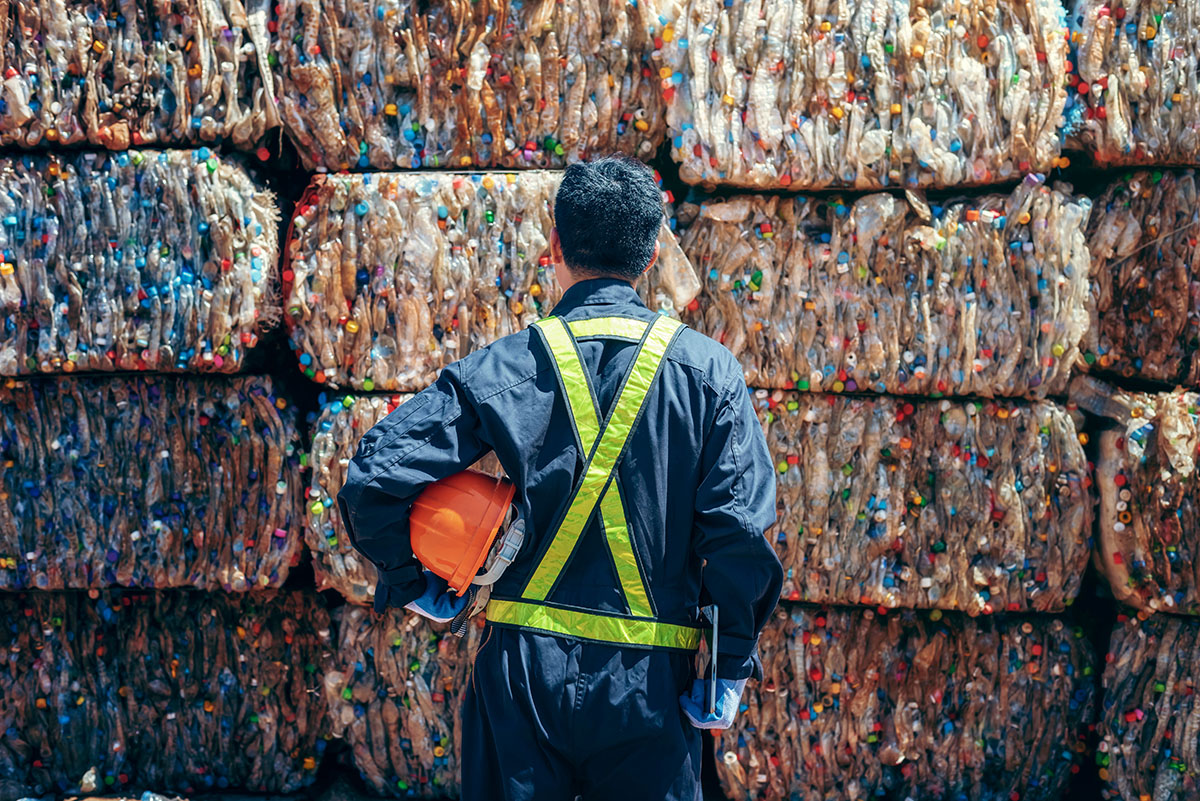
A data-driven approach can promote adaptability by predicting market demand and recycling rate uncertainty.
Fluctuations in recycling rates and market demand for recycled materials can lead to unpredictability for plastic manufacturers. To combat this issue, researchers investigated how historical data can improve the responsiveness of plastic production. Researchers combined a Rolling Horizon (RH)–based Mixed Integer Linear Programming (MILP) model with a rolling Long Short-Term Memory (LSTM) model. Using this approach, researchers found up to 80% reductions in production costs. Additionally, this technology can resolve challenges with material inventory and late orders during distribution.
You can also read: Polyurethane (PU) Recycling: Growing Challenge and Innovation.
A Dynamic Solution to Changing Markets
Previously, many studies used static approaches when tackling production planning problems. These approaches assume that input data will already be available, and thus, researchers plan the entire solution at once. Outside of a simulation, this is often not the case. When reality differs from optimality, manufacturers can accrue significant costs by not adjusting to real-time changes.
When a manufacturer orders raw materials, their decision is irrevocable. Buyers calculate the quantity of plastic waste ordered based on the forecasted recycling rate. Raw material inventory levels influence holding costs, and recycling rate and future demand influence production scheduling and raw material sourcing. Additionally, market demand influences the backlog and inventory levels. These many moving parts are essential to ensure manufacturers meet demand and accommodate production capacities.
Instead of planning in the long term, RH approaches suggest a series of shorter-term planning horizons. As each horizon ends, the algorithm updates the plan with the latest information, which updates the forecast. This process iterates for each horizon, helping to plan for sudden changes in market conditions or unexpected events. The novel algorithm can tailor production to the current market demand and recycling rate, even when these fluctuate.
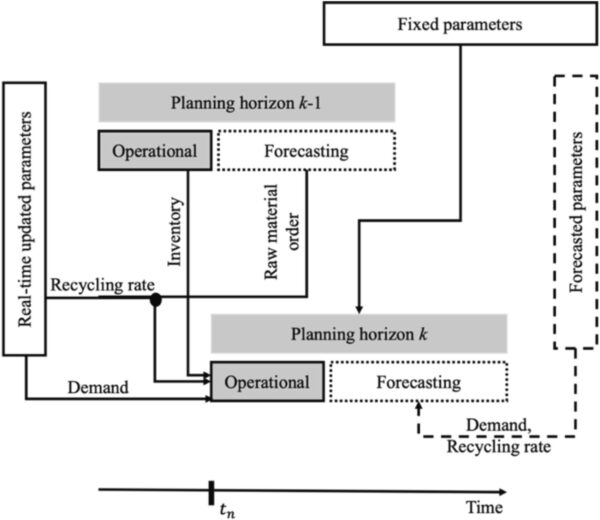
Parameters updated in real-time can ensure each planning horizon uses the most current data. Courtesy of A novel data-driven rolling horizon production planning approach for the plastic industry under the uncertainty of demand and recycling rate.
Planning for the Future
To assess the outputs of this system, researchers performed a sensitivity analysis. Researchers tested various rolling durations for backlog, raw material and final product inventory, and late order. Results showed that, of these factors, increasing rolling duration most significantly affected raw material inventory. Shorter rolling durations, or more frequent system updates, can minimize costs associated with this.
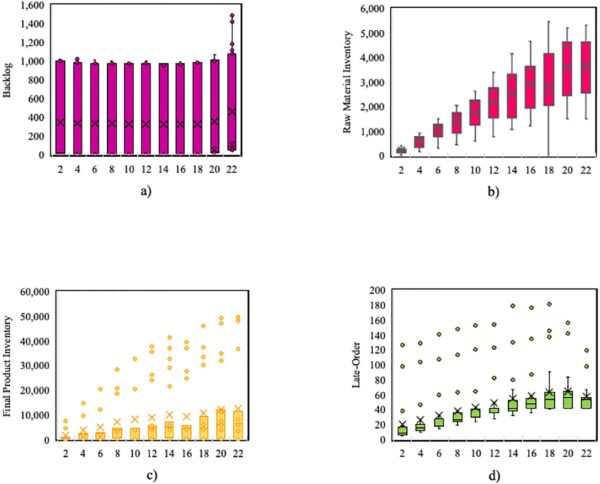
The impact of rolling duration on a) backlog, b) raw material inventory, c) final product inventory, and d) late order. Courtesy of A novel data-driven rolling horizon production planning approach for the plastic industry under the uncertainty of demand and recycling rate.
The recycling industry is a constantly changing landscape. When manufacturers intend to use recycled materials in their products, adaptability is necessary. Algorithms such as this combined RH and LSTM-based model can help accommodate sudden changes and minimize production costs. This study demonstrated that the novel approach had superior performance compared to the static counterpart. Going forward, additional research could help expand the planning framework for distribution centers and customers.